Clinicians should know that artificial intelligence (AI) in radiology is an evolving field with significant promise, but also with certain limitations and ethical considerations. AI applications mainly focus on image recognition, risk assessment, and data management, with the aim to enhance diagnostic accuracy, streamline workflow, and improve patient care. These systems, however, are not infallible and their performance can vary based on the quality and diversity of the data they were trained on.
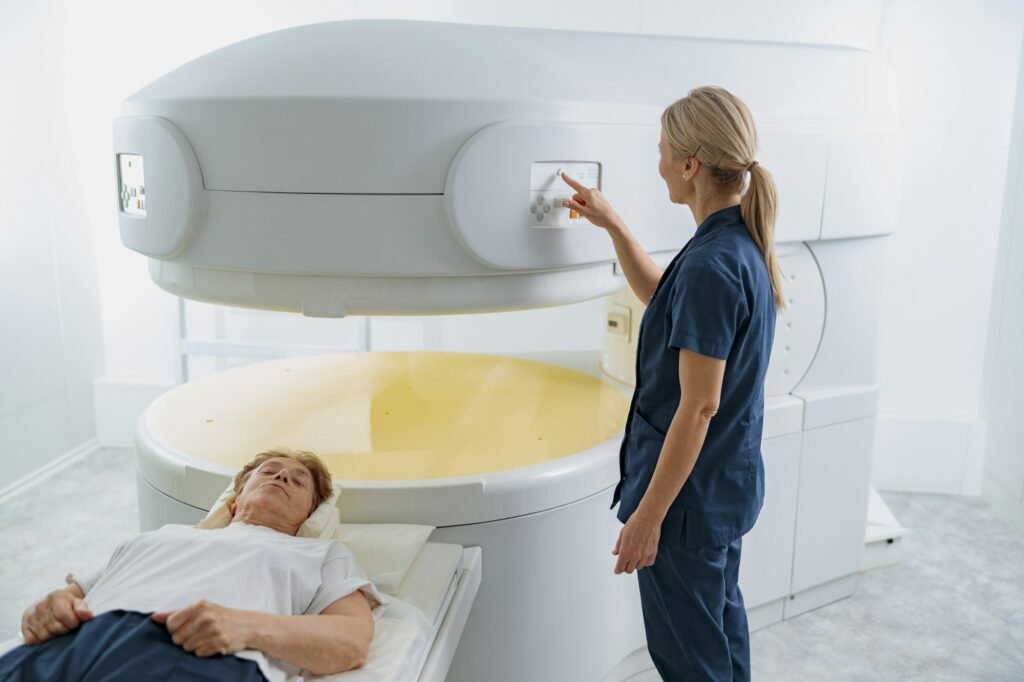
Therefore, clinicians should consider AI as a supplementary tool rather than a replacement for human expertise. Additionally, there are important ethical and legal considerations, including data security, potential biases in AI algorithms, and accountability in case of errors. Finally, it is important for clinicians to engage in continuous education and training on AI tools, as these systems evolve rapidly. Understanding these aspects will help clinicians to develop an informed trust in the application of AI in radiology and to optimize its use in patient care
Basics about AI for clinicians
Supplemental Tool
AI is used as a supplemental tool to assist radiologists in interpreting medical images, not as a replacement for human expertise.
Diagnostic Accuracy
AI applications can enhance diagnostic accuracy by helping identify patterns and abnormalities in medical images that may not be immediately visible to the human eye.
Workflow Efficiency
AI can streamline workflow by automatically analyzing images, thus reducing the workload of radiologists and speeding up the diagnostic process.
Risk Assessment
AI applications can assess the risk of certain conditions by analyzing medical images and clinical data.
Algorithm Training
AI algorithms are trained using large datasets of medical images and then tested and validated to ensure their efficacy and safety.
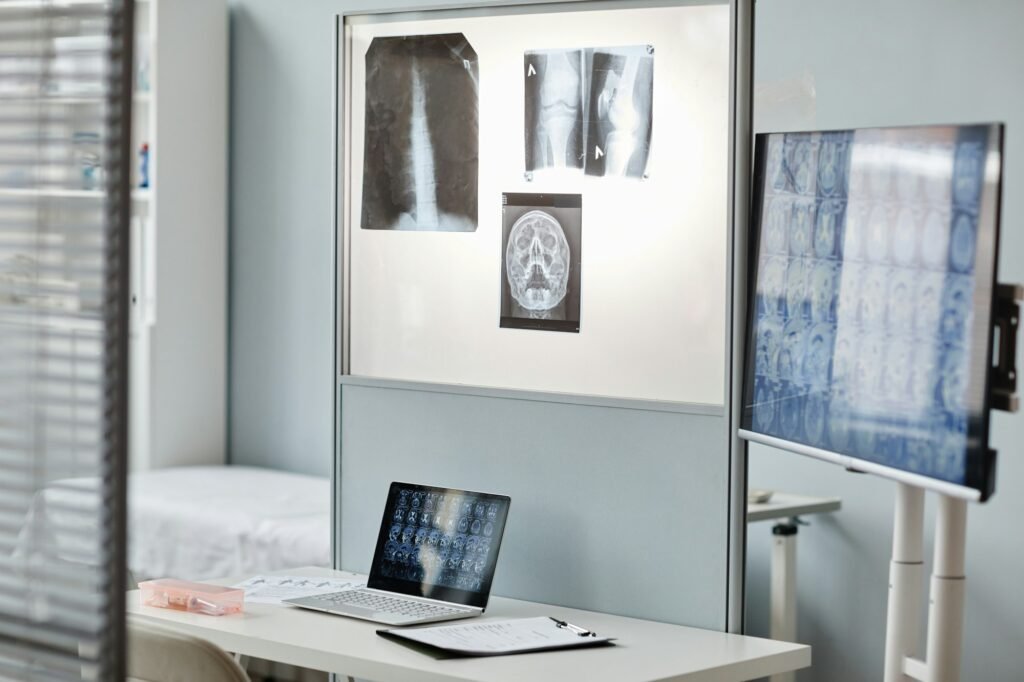
Ethical and Legal Considerations
There are important ethical and legal considerations, such as data security, potential biases in AI algorithms, and accountability in case of errors.
Continuous Education: Clinicians and radiologists need continuous education and training on AI tools as these systems evolve rapidly.
Data Security
Ensuring the security of patient data is crucial when using AI applications in radiology.
Potential Biases
AI algorithms can have potential biases based on the data they were trained on, which needs to be considered when interpreting the results.
Accountability
There should be clear accountability in case of errors or misdiagnoses made by the AI system.